When gastronomy becomes gastrostomy: The dangers of machine translation typos
Programs that facilitate the understanding of texts and dialogues in another language can replicate, replace, or overlook original errors, which can have significant consequences in legal, medical, or conflict-zone settings
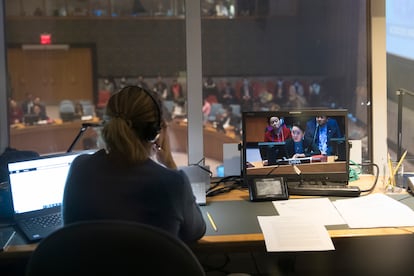
Almost all translation professionals defend their work against automatic tools by recalling notable errors, such as the now infamous promotion on the city of Santander’s website. This site, translated mechanically without human oversight, famously turned references to the Botín Centre (named after the prominent local financial family) into the “Loot Center,” and mistranslated the historic quarter (casco viejo) as “historic helmet.”
A recent study by Santiago Rodríguez-Rubio, a professor at Pablo de Olavide University (UPO) in Spain, highlights another issue with common machine translation tools: typos. These errors may be replicated, replaced, or ignored altogether, posing serious risks in critical fields like medical, financial, legal, or conflict-zone communications.
Stephen Ibaraki, an international technology consultant and founder of AI For Good, illustrates the positive side of such technology: “An Indian farmer, unable to speak all 22 official languages, can now communicate their needs using just a phone. In Africa, where AI is gaining ground, ordinary people can access medical help or enhance their financial inclusion. Meta’s latest smart glasses have simultaneous translation capabilities, a feature expected to become widely available.”
However, in complex scenarios, everyday applications reveal vulnerabilities. Fourteen years ago, the U.S. Defense Advanced Research Projects Agency (DARPA) launched the Transtac project, aimed at developing a translation system for tactical use in 25 conflict scenarios, including checkpoint interactions, key information exchange, and medical assessments. While the system achieved 80% accuracy — considered quite good — it fell short for use in delicate situations where a mistake could lead to violence.
Medical translations also demand heightened precision. Researchers from the universities of Michigan, New York, and Washington analyzed OpenAI’s Whisper application and found hallucinations (mistakes or fabricated sentences presented as accurate) in 38% to 80% of audio-to-text cases. Whisper — used for transcribing meetings and creating subtitles — has also found application in medical centers for recording patient consultations.
Such mistakes could have “really grave consequences,” particularly in hospital settings, Alondra Nelson, a Princeton University professor and former director of the White House Office of Science and Technology Policy, told the Associated Press. “Nobody wants a misdiagnosis. There should be a higher bar.”
In one transcript reviewed, Whisper invented a nonexistent drug called “hyperactivated antibiotics.” OpenAI acknowledged these findings, emphasizing that while the company is working on reducing hallucinations, the tool should not be used in “high-risk domains.”
The same risks apply in legal and financial settings, where precise language is crucial. Rodríguez-Rubio cites an instance where an expression meaning “excess of liabilities” was mistakenly rendered in English as “axes” of liabilities.
To test machine translation systems, Rodríguez-Rubio introduced 1,820 typographical errors into source texts previously used in specialized Spanish-English dictionary studies. The analysis revealed that “repetition of the original typo is the most frequent phenomenon” across two systems tested: Google Translate and DeepL. While Google Translate performed better, both systems exhibited deficiencies, such as replicating typos (e.g., the typo excusive was translated as escusivo), deleting errors, or transforming words (e.g., if vacancies was accidentally written as vancies, it was turned into vans).
Rodríguez-Rubio, a UPO doctor and member of the Department of Philology and Translation, does not oppose automatic translators, quite the opposite. His research is meant to “illuminate the capacity of these systems to manage typographical errors in source texts and provide a starting point for their improvement.”
“AI’s applications in language correction are undeniable. Technology has always been, and will continue to be, a fundamental pillar, though this does not preclude examining its potential drawbacks — such as information noise, immediacy culture, blind trust, and reduced reflection,” he notes. The human element, he emphasizes, remains crucial.
For Rodríguez-Rubio, the spread of typographical errors “is a symptom of a deeper problem.” “The typos that plague modern texts are an indicator of the underlying problem of the dysfunctions of the technological revolution, of the reconfiguration of the role of man and machine in work processes, of the culture of haste and immediacy,” he says.
While the study’s “limited scope” focuses on how original errors affect the translation of isolated words or short phrases, it suggests ways to enhance AI systems. He proposes further exploration into synonymy (e.g., rejection versus refusal), antonymy (such as DeepL’s translation of undestanding as without understanding), and paronymy (detecting near-homonyms like gastronomy versus gastrostomy).
The success of machine translators hinges on their data sources — the backbone of any AI application. If source errors are perpetuated or worsened, system accuracy falters. “A strong data foundation is at the core of generative AI capabilities,” cautions Baris Gultekin, AI lead at Snowflake, in the presentation of a study conducted in collaboration with MIT Technology Review Insights.
Supporting this, research in Nature shows AI results degrade when trained on AI-generated data. Ilia Shumailov of the University of Oxford likens the process to repeatedly copying a photo. “If you take a picture and you scan it, and then you print it, and you repeat this process over time, basically the noise overwhelms the whole process,” he says, leading to what he terms “model collapse.”
Google is aware of these challenges and is working to address them. A spokesperson stated: “Translate learns patterns from millions of examples online. Unfortunately, these patterns sometimes yield incorrect translations. We value user feedback for quick corrections and rigorously train and test our systems to uphold quality across all our tools.”
Sign up for our weekly newsletter to get more English-language news coverage from EL PAÍS USA Edition
Tu suscripción se está usando en otro dispositivo
¿Quieres añadir otro usuario a tu suscripción?
Si continúas leyendo en este dispositivo, no se podrá leer en el otro.
FlechaTu suscripción se está usando en otro dispositivo y solo puedes acceder a EL PAÍS desde un dispositivo a la vez.
Si quieres compartir tu cuenta, cambia tu suscripción a la modalidad Premium, así podrás añadir otro usuario. Cada uno accederá con su propia cuenta de email, lo que os permitirá personalizar vuestra experiencia en EL PAÍS.
En el caso de no saber quién está usando tu cuenta, te recomendamos cambiar tu contraseña aquí.
Si decides continuar compartiendo tu cuenta, este mensaje se mostrará en tu dispositivo y en el de la otra persona que está usando tu cuenta de forma indefinida, afectando a tu experiencia de lectura. Puedes consultar aquí los términos y condiciones de la suscripción digital.