Why is AI unable to predict disasters like Spain’s flash flooding in time?
The technology’s ability to forecast floods is limited by the poor quality of data and the irregular behavior of weather events such as a DANA
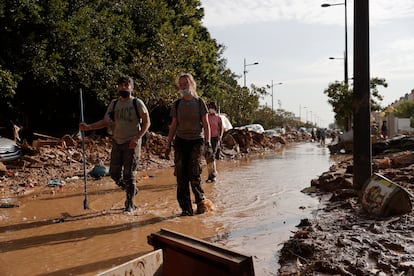
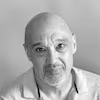
Artificial intelligence (AI) is already capable of identifying tumors from thousands of images, performing precise surgical operations, discovering new antibiotics and proteins, analyzing market trends, and optimizing industrial processes, among numerous other applications. Yet, when it comes to adverse phenomena like floods — the most destructive natural disasters after earthquakes and tsunamis — its technological prowess falls short.
Over the past two decades, floods have impacted 2.5 billion people, claimed the lives of a quarter of a million individuals, and caused an estimated $936 billion in damages, according to the International Disaster Database (EM-DAT) from the Catholic University of Louvain in Brussels. Despite the focus of technological giants and global institutions, developments to predict and mitigate floods remain ineffective. Why is that the case?
“Existing climate models aren’t very good for certain extreme weather events, Heatwaves in Europe are, for example, increasing much faster in the real world compared to what the models tell us should happen,” Dim Coumou, a climate expert at the University of Amsterdam, told Horizon magazine. “It’s important to forecast extremes so we can have early warnings.”
One major obstacle to accurate forecasts is the data available to train artificial intelligence models. While historical data spanning decades is available, it may not be relevant when it comes to understanding the most adverse phenomena. “Extreme events are, by definition, rare. So you don’t always have many observations. That is a major hindrance if you want to use AI methods,” Coumou explained.
AI generates results by identifying patterns derived from analyzing vast amounts of data. For instance, it can recognize a tumor in an image if it has thousands of comparable, high-quality images supported by confirmed diagnoses.
AI needs masses of high-quality data to be useful for science, and databases containing that sort of data are rare, said David Baker, who won the Nobel Prize in Chemistry this year for developing open artificial intelligence systems that create new proteins.
The lack of data on rare phenomena is further exacerbated by the difficulty of accurately predicting meteorological events as dynamic and complex as a DANA (isolated depression at high levels), which was responsible for the deadly flash flooding in Spain last week. A DANA causes intense rainfall and storms that can be highly localized and vary in intensity. Its behavior is erratic, influenced by a multitude of factors, including ambient temperatures, humidity, pressure, wind patterns, topography, and interactions with other geographic and atmospheric elements.
Even long-term data does not guarantee the ability to precisely predict when and where a storm will strike. Moreover, even if this data were to reveal a consistent rainfall pattern, it would still fall short in resolving the inherent uncertainties surrounding these events. “Very intense storms do not automatically result in damage,” Kevin Collins, professor of environment and systems at the Open University in the United Kingdom, told Science Media Center.
Professors Víctor Resco de Dios and Domingo Molina from the University of Lleida in Spain agree. “It is not enough to know how much and where it will rain, but we also need to establish how that rain will turn into floods and which areas will be potentially affected,” they write in The Conversation.
The fleeting variability of all the factors involved, along with the dispersion and scarcity of specific data on extreme weather phenomena, and the heterogeneous quality of available data, significantly limits artificial intelligence in identifying patterns and generating effective prevention and alert systems. Developing a forecasting system with a lead time of just 24 hours, according to the Global Commission on Adaptation, “would reduce damage by 30%.” A study published in Nature Communications estimates that this would translate to an annual savings of $143 billion, which exceeds the average damage reported by EM-DAT when factoring in human losses.
The key is not just the reliability of the prediction, but also the lead time. “No matter how accurate a forecasting is, its forecast does not have any value as information if it does not come soon enough,” said Hwang Seokhwan, a researcher at the Korea Institute of Civil Engineering and Construction Technology (KICT) who is working on a flash flood forecasting system.
Despite the challenges, technology giants such as Google and IBM, in collaboration with NASA and various European and global institutions, are working within the framework of the United Nations’ five-year plan to develop artificial intelligence tools that can provide reliable medium- and long-term predictions, as well as improve early warning systems.
Google DeepMind, the AI subsidiary of the American tech giant, unveiled a weather forecasting model in Science that uses machine learning to make “better, faster, and more accessible 10-day weather predictions,” according to the study. The model, named GraphCast, outperformed traditional forecasting systems in 90% of the cases tested.
The system used as a reference was the European Centre for Medium-Range Weather Forecasts (ECMWF), which operates a supercomputer in Bologna, Italy, equipped with around one million processors and 30 petaflops (30,000 trillion calculations per second) of capacity. This center, which integrates AI in its Integrated Weather Forecast System (AIFS), accurately predicted the torrential rains that struck central Europe in September.
GraphCast does not require the immense computational power of traditional weather forecasting systems. Instead, it relies on machine learning trained on historical data to deliver accurate 10-day forecasts in under a minute. “We believe this marks a turning point in weather forecasting,” write the authors, led by DeepMind scientist Remi Lam.
IBM is also in the race, collaborating with NASA on a machine learning-based proposal. “AI foundation models utilizing geospatial data can be a game-changer because they allow us to better understand, prepare and address the many climate-related events effecting the health of our planet in a manner and speed never before seen,” said Alessandro Curioni, IBM Fellow and Vice President, Accelerated Discovery at IBM.
According to Kate Royse, director of the British supercomputing center Hartree, these models “will enable smarter decisions based on accurately predicting and managing flood risk, which is critical to all future city planning.”
“A good use of the AI-based weather forecasts would be to complement and enhance our forecasting toolbox, perhaps by allowing us to produce larger ensembles of forecasts that enable accurate assessment and interpretation of the likelihood of extreme events,” Andrew Charlton-Perez, a professor of meteorology at the University of Reading in the United Kingdom, told Reuters.
A complementary system is also being investigated by two centers of the German Helmholtz scientific consortium, with results published in Nature Communications. This model combines precipitation forecasts from the weather service with data on soil moisture — one of the key factors in flood development — and flow rates. It aims to predict flood zones, depths, and potential damage to critical infrastructure, such as buildings, streets, railways, and hospitals.
“The responsible authorities and the population therefore not only have information on a possible gauge water level 30 kilometers [18.6 miles] upstream, but also a high-resolution flood map showing the impacts of the flood. For example, they could know where people could be in danger or who has to be evacuated,” said hydrologist Sergiy Vorogushyn from the German Research Centre for Geosciences and co-author of the study. “For example, they could know where people could be in danger or who has to be evacuated.”
Sign up for our weekly newsletter to get more English-language news coverage from EL PAÍS USA Edition
Tu suscripción se está usando en otro dispositivo
¿Quieres añadir otro usuario a tu suscripción?
Si continúas leyendo en este dispositivo, no se podrá leer en el otro.
FlechaTu suscripción se está usando en otro dispositivo y solo puedes acceder a EL PAÍS desde un dispositivo a la vez.
Si quieres compartir tu cuenta, cambia tu suscripción a la modalidad Premium, así podrás añadir otro usuario. Cada uno accederá con su propia cuenta de email, lo que os permitirá personalizar vuestra experiencia en EL PAÍS.
¿Tienes una suscripción de empresa? Accede aquí para contratar más cuentas.
En el caso de no saber quién está usando tu cuenta, te recomendamos cambiar tu contraseña aquí.
Si decides continuar compartiendo tu cuenta, este mensaje se mostrará en tu dispositivo y en el de la otra persona que está usando tu cuenta de forma indefinida, afectando a tu experiencia de lectura. Puedes consultar aquí los términos y condiciones de la suscripción digital.