Google AI identifies millions of protein mutations capable of causing disease
Only 2% of known variations had been classified, but DeepMind’s artificial intelligence has increased that figure 18-fold
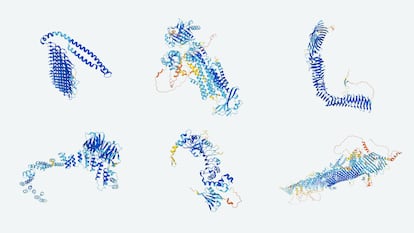
It’s the holy grail of modern medicine: identifying the alterations in the genome that lead to genetic diseases. It’s no easy task: each individual has thousands of mutations in the genetic information they inherited from their parents. Most are benign, but there is a percentage that can be pathogenic. Now, researchers at Google DeepMind, Alphabet’s artificial intelligence company, have cataloged 71 million of these mutations. The program was also able to classify their level of risk. It found that a third could modify the functioning of the proteins, causing serious diseases.
DNA contains the instructions for the development of all living beings. This book contains each of its recipes for creating cells, organs and functions in the form of the sequences of their basic components. These basic components, the building blocks of life, are proteins. They are made up of a series of amino acids, sometimes hundreds of them, which are made up of trios of nucleotides, the letters of the genetic alphabet. When one of these nucleotides is replaced by another in a mutation, it is called a missense variant. For the most part, these variants do not affect the function of the protein. But in other cases, the mutation is catastrophic, degenerating into pathologies such as amyotrophic lateral sclerosis (ALS) or sickle cell anemia.
Until now, about 4 million of these missense variants had been identified in the 19,233 proteins that make up each human being. But only 2% of cases had been identified as either benign (the majority) or the possible source of diseases. Now, artificial intelligence (AI) has increased that number 18-fold, classifying most of them by potential impact on protein function.
The authors of this achievement, published in the prestigious scientific journal Science, are DeepMind scientists. A few years ago, this same group developed AlphaFold, an AI program that was able to predict the structure of practically all known proteins — a feat considered one of the greatest advances in computational biology. For this new project, the researchers redesigned and reoriented the program to detect missense mutations in protein expression. What’s more, in its training, the new tool, AlphaMissense, was able to classify with high probability the impact that the variant may have on the function of the protein.
AlphaMissense
DeepMind researcher Jung Chen, the lead author of the study, explains how AlphaMissense works: “We knew that AlphaFold was a very good model for predicting the three-dimensional structure of proteins from a massive sequence. We also knew that the 3D structure of proteins is very important for their function, basically revealing what it is,” explains Chen. If its function can be deduced from the structure, any alteration in that structure could be the result of a mutation. Another fundamental piece is AlphaMissense’s ability to learn from the evolutionary limitations of related sequences. In other words, the researchers were able to gain insight from the fact that evolution has shaped what the structure of a protein should and should not be like. To improve its knowledge in this area, the system was trained with the structures of human and primate proteins. “Through training, you see millions of protein sequences and learn what a normal protein sequence looks like. And when we are given one with a mutation, it can tell us if it is bad or not,” he adds.
“It’s very similar to human language,” he explains. “If we substitute a word in an English sentence, a person who is familiar with the language can immediately see whether the substitute will change the meaning of the sentence or not.” AlphaMissense was able to classify 89% of the 71 million missense variants it identified. Of them, 57% were likely benign and a third were likely pathogenic. Of the remaining 11%, AI was unable to classify the impact. “The model assigns a score between zero and one to each of the variants and indicates the probability that the variant is pathogenic. By pathogen, we mean that our pathogenic variant is more likely to be associated with or cause a disease,” says Chen.
Chen’s explanation highlights both the strength of AlphaMissense — its high capacity to classify variants — and one of its weaknesses — the percentages are just probabilities. Until the era of powerful computers and AI, characterizing the structure of a protein and its mutations, was a titanic job. Before the arrival of these technologies, researchers determined the structure of some 200,000 proteins, a task that took 60 years and thousands of scientists. The milestone required many hours in the lab and the use of particle accelerators. But these were real observations of real protein structures. In the case of computational biology, they are virtual proteins and variants, which must then be confirmed. In the case of AlphaMissense, its calculations are 90% accurate.
“Understanding the disease”
Regarding possible applications, Žiga Avsec, the senior co-author of the study who is also from DeepMind, said in an online conference that the first step in finding treatments is understanding the disease. “For both complex and rare diseases, that means finding the genes associated with them,” he explained. For Avsec, tools like AlphaMissense can help better identify variants and discover potentially new genes. By better understanding genetics, researchers will be able to form stronger opinions about whether particular genes are related to diseases, he said. According to Avsec, the path towards finding treatments lies in “better genetics, finding new genes and gaining greater statistical power to detect new associations.” He, however, said that this will not directly lead to new drugs.
A few days ago, the analysis of the 200 million proteins discovered by AlphaFold last year was made public. Spanish bioinformatician, Íñigo Barrio, who took part in the projects, says “AlphaFold changed the world.” But he is less enthusiastic about AlphaMissense. “It is important, it is a new way to evaluate variants, and it could be used to monitor rare diseases. But there are other prediction softwares that already exist,” he says, Barrio also highlights one of the limitations of the AI tool. AlphaMissense catalogs missense variants individually, but many genetic disorders “are the product of the combination of several of these mutations,” he explains.
Biologist José Antonio Márquez, who directs the Crystallography Platform of the European Molecular Biology Laboratory, agrees: “It is one of the applications of the [AlphaFold] method, it may not be so relevant at a scientific level, but it is relevant when it comes to starting to transfer a discovery into possible applications.” Among these applications, Márquez highlights its use to accelerate “research in genetic diseases, particularly rare diseases, since it helps generate hypotheses about the mechanism that causes the disease.”
Sign up for our weekly newsletter to get more English-language news coverage from EL PAÍS USA Edition