Pushmeet Kohli, Google DeepMind: ‘Our next challenge is to understand the semantics of DNA’
The director of the corporation’s scientific laboratory points to genomics, nuclear fusion and the development of new materials as future areas of exploration for his team
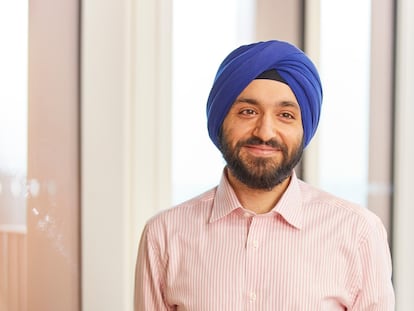
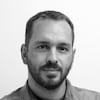
Artificial intelligence has become a fundamental tool of scientific advancement. “As a computer scientist, I believe that the human being is the most complex program ever written. It’s amazing to be able to access a piece of that code,” says Pushmeet Kohli, vice-president of research at Google DeepMind. He knows what he’s talking about. His boss, Demis Hassabis, and colleague John Jumper won the Nobel Prize in Chemistry for their contribution to “protein structure prediction” using artificial intelligence. The award recognizes the utility of AlphaFold2, their tool which has succeeded in describing the three-dimensional shape of 200 million proteins, key information for the understanding how organisms function.
According to Kohli, AI has opened up new horizons. “In any area of science you look at, AI is transforming what can be done,” he tells EL PAÍS after speaking at the AI for Science forum that was hosted in London by his company and the Royal Society.
Question. Is there any scientific discipline that cannot benefit from the advancement of AI?
Answer. If you can formulate the scientific question you are working on as a reasoning problem or as a pattern recognition problem in which certain conclusions have to be drawn from the data, then AI has a lot to contribute. A common mistake is to forget that you have to be able to capture the data from the physical objects you are studying. For example, there is no point in making models that try to predict emotions, because the data you will train it with are subjective reactions of humans who have seen this or that facial expression or body language in given contexts. It is very important for us to know the limitations of these models.
Q. What kinds of projects is your team interested in?
A. We have a lot of work around biology. We have touched on structural biology, but we are also very interested in genomics: we want to understand the semantics for DNA, to know what happens with the problems involving variants of unknown significance. That’s our next challenge. If there is a mutation in the genome, what specific effect does it have? We are also working on new materials, we believe they hold a lot of potential for progress. Other important areas for us are nuclear fusion, climate, and basic science related to mathematics and informatics.
“Congratulations to John, the #AlphaFold team, and everyone at DeepMind & Google that supported us along the way - it’s an amazing award for all of us! It’s such an honour and privilege to work with all of you to advance the frontiers of science.” - @DemisHassabis
— Google DeepMind (@GoogleDeepMind) October 10, 2024
Find out more… pic.twitter.com/XAr86gFEf3
Q. What are your objectives when it comes to fusion and new materials?
A. Within nuclear fusion, the objective is to maximize the time we can maintain plasma stability. When the fusion reactor is turned on, our AI system controls the magnetic field, which has to be subtly modulated without causing disruptions that destabilize the plasma, maintaining the right temperature and friction. In terms of the development of materials, the goal is to develop new materials that, when tested in the laboratory, we see are synthesizable and stable.
Q. You said that in the area of genomics, the goal is to understand the semantics of DNA. At what point are you at in that process?
A. The Human Genome Project sequenced three billion letters in the code that makes us who we are. It turns out that all those letters have a meaning, a purpose that we currently don’t fully understand. There are two components of the genome: the coding part and the non-coding part. The former speaks to which proteins will be expressed, the latter about the regulatory mechanisms related to how much protein should be expressed, and so forth. For the coding part, we are already making predictions with a high level of reliability. We believe we are close to being able to say whether or not certain mutations are going to be problematic. But knowing how and why they will be problematic is still an open area of research. And the same goes for the non-coding part. We want to know how protein expression happens. There is no horizon for finishing the project right now. But, when we do, we will truly have an understanding of the language of life. And then, we can start thinking about how to edit the genome to achieve certain goals.
Q. To what extent has the race for generative AI, which in Google’s case is against Gemini, distracted from other lines of research at your company?
A. Generative AI is a very powerful concept, including for science, because it has unlocked something new. Up until now, a large part of our efforts focused on taking advantage of data that was structured, in the sense that you have a sequence and a prediction, you could see the results in tabular form. Now, a lot of scientific breakthroughs are presented in text-based papers, so we were not able to apply AI to take advantage of the sort of intuition it brings. Large language models have allowed us to extract knowledge from that scientific literature. So in a sense, generative AI is helping in science because it is opening up a new field.
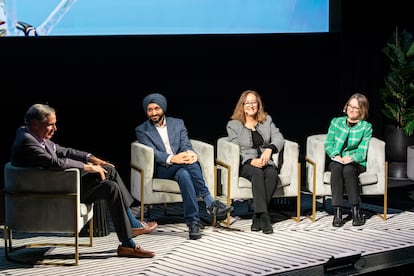
Q. Generative AI is supported by gigantic databases, which have already exhausted the entire internet. It’s starting to be said that the next models will be trained with synthetic data created by machines. What’s your take on this?
A. I think that the bigger a model, the more expressive it is and the greater level of freedom it has. With more data, we can have more supervision and control over what the system is going to learn. But that is not a question of size, what is really important is the diversity of the data, which contributes different kinds of problems to the models from which they can learn.
Q. Will you achieve that with synthetic data?
A. It’s not something that works in all cases. Normally, we use data that has been obtained by carrying out experiments. In the case of AlphaFold, it was trained on a database of 150,000 proteins and, through that training, we were able to predict the structure of more than 200 million. In some cases, we used simulations. We do this in our nuclear fusion projects: we try to see possible ways in which the plasma can behave in order to control it, with the idea that, when applied to a nuclear reactor in the real world, the system will be able to generalize. And finally, there is the idea of AI-generated synthetic data. In some cases, you can have the model produce certain types of data that were not present in the training database. For example, let’s imagine that in the original database we only have images of green chairs, but in a synthetic database, since we know the concepts of blue and red, we generate chairs of many different colors. The final model will be able to understand that chairs can be of various colors and detect them.
Q. What kind of problems can be solved with this kind of model?
A. It can be applied to nearly any problem we can imagine, but may not work with all of them. We still don’t have a theory to understand when synthetic data is useful. But in some cases, we’ve proven that by using this technique we get an improvement in the system’s performance.
Q. What proportion of synthetic data do you use?
A. We are investing in these three kinds of data sources. Especially in simulation, which is the most effective source and can be controlled. We are using synthetic data, but cautiously: it’s very important for the original model to be good because if it isn’t, its results won’t be useful.
Sign up for our weekly newsletter to get more English-language news coverage from EL PAÍS USA Edition
Tu suscripción se está usando en otro dispositivo
¿Quieres añadir otro usuario a tu suscripción?
Si continúas leyendo en este dispositivo, no se podrá leer en el otro.
FlechaTu suscripción se está usando en otro dispositivo y solo puedes acceder a EL PAÍS desde un dispositivo a la vez.
Si quieres compartir tu cuenta, cambia tu suscripción a la modalidad Premium, así podrás añadir otro usuario. Cada uno accederá con su propia cuenta de email, lo que os permitirá personalizar vuestra experiencia en EL PAÍS.
¿Tienes una suscripción de empresa? Accede aquí para contratar más cuentas.
En el caso de no saber quién está usando tu cuenta, te recomendamos cambiar tu contraseña aquí.
Si decides continuar compartiendo tu cuenta, este mensaje se mostrará en tu dispositivo y en el de la otra persona que está usando tu cuenta de forma indefinida, afectando a tu experiencia de lectura. Puedes consultar aquí los términos y condiciones de la suscripción digital.