Recordings of a one-year-old’s life train an AI system to learn words
Research establishes a computational basis to study how children begin to speak, connecting what they see with the auditory stimuli they receive from adults
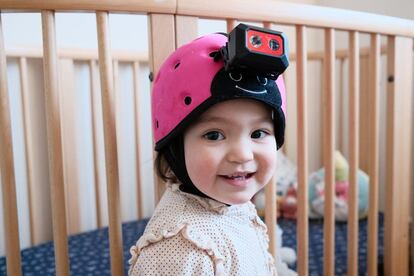
From birth, babies begin to receive visual and auditory stimuli, essential to learning language. Between the ages of six and nine months, most infants begin to talk, associating sounds with real-world objects and concepts. By the time they reach the age of two, they usually have a vocabulary of approximately 300 words. But how does this learning process develop?
A team of researchers from New York University studied recordings of a child’s daily routine during their first year of life to find the answer. The experiment not only confirmed the connection between visual and linguistic representation — that is, what is seen and the word that corresponds to it — but also contributed to the development of an artificial intelligence (AI) model, which has managed to recognize different objects in a similar way to how children do.
“Large AI systems are trained and powered by an astronomical amount of data. We’re talking about [huge amounts of] words to be able to develop a language system,” explains Wai Keen Vong, a professor of psychology and computer science, who coordinated the study that was published in the journal Science this past week. “However, humans need only a few thousand words to achieve an efficient communication system,” he clarifies. Out of this contrast, there was interest in investigating whether an AI model would be able to learn to speak in the same way that children do: by observing their environment, listening to the people around them and connecting dots between what they see and hear.
Early language acquisition is a widely-debated topic: several hypotheses about how it occurs have been proposed. Traditionally, these types of studies have been conducted in controlled laboratory settings, resulting in discoveries that often don’t extrapolate effectively to more dynamic and varied real-world contexts. “The novelty of this analysis lies in the fact that we were able to work with first-hand data, derived from a real learning situation,” Dr. Vong emphasizes.
To this end, his team analyzed 61 hours of the life of Sam, an Australian boy who, for a year-and-a-half — from the age of six months to 25 months — wore a helmet with a camera that recorded the interactions he had with his parents and grandparents on a daily basis. In reality, he recorded only 1% of the time he spent awake during the duration of the experiment. Even so, hundreds of images have been achieved that reproduce exactly what the child was seeing, accompanied by the linguistic expressions of his family members, who explained the nature of the objects that surrounded him. “For example, during mealtime, the camera on his head recorded the image of a spoon, at the same time that his mother asked him something related to that utensil. And so on, with dozens of everyday objects,” Vong recalls.
The connection between these two mediums is almost never obvious. In fact, the researcher recognizes that part of the challenge for babies is to understand exactly what word is associated with the object that they’re interacting with. “Most of the time, parents aren’t labeling every object. For every ball Sam was looking at, his parents didn’t tell him ‘this is a ball’ [or] ‘look at the ball.’ He listened to the words in a natural context. The difficulty [for a child] is to find out — within a relatively long sentence — which word corresponds to the round object that they’re playing with,” Vong points out.
Training AI like a baby
After observing the child’s behavior, the researchers were able to confirm that he learned the meaning of the words by connecting the visual stimulus — that is, the image presented to him — with the response of his family members, who repeated the corresponding word. With these results, they’ve moved on to the second phase of the experiment: verifying whether an AI system would be able to learn to recognize objects in the same way that Sam did.
The artificial intelligence model, called CVCL (Child’s View for Contrastive Learning) has been trained with 64 visual categories — utensils, toys and animals, among others — and the transcription of what Sam was hearing while looking at these objects. Once this database was created, the researchers began testing to see if the AI was capable of identifying the images. According to Vong, the model — with limited sensory information and relatively generic learning mechanisms — can provide a computational basis for investigating how children acquire their first words and how those words can connect to the visual world.
“We found that CVCL can learn to make connections between images and text from limited fragments of a single child’s experience,” the authors highlight in the study. In some cases, the objects appear on a white background, while in others, they’re in an environment with more stimuli. In fact, the model’s classification accuracy was 61.6%. It remained high even when images other than Sam’s recordings — on which the AI had not been trained — were inserted into the system. “The results confirm our hypothesis that with only two impulses — which are what the child sees and what they hear — it’s possible to achieve and accelerate this type of learning,” Vong highlights.
Studying how speech is born
Antonio Rodríguez Fornells — a researcher at the Institute of Neurosciences of the University of Barcelona — points out the novel aspect of the study, which paves the way to understanding (via computational simulations) what are the minimum learning mechanisms that children use to face the challenge of learning a language: “Previous studies on babies in developmental psychology provide key information with very novel experiments, but the lack of neuroscience or neuroimaging studies on them (due to the difficulty of applying these techniques to babies) doesn’t allow for much progress. [It’s difficult to] clarify the brain mechanisms that support these language acquisition processes,” this neuroscientist explains.
He points out that the simulations proposed in the article support certain previously proposed theories of language. “Among them, that the simple associative learning mechanism (that allows for the linking of images and words) in a natural learning environment (such as that experienced by children in the first months of their life) is enough to be able to comprehend these relationships and generalize the content of meaning,” Rodríguez Fornells adds.
Even so, the study has some limitations. The CVCL model was trained with recordings from a single head-mounted camera, placed on a single child. And, rather than direct speech, speech transcriptions were utilized in the training: these omit important nuances, such as intonation and emphasis. “It must also be remembered that the model’s learning [process] was passive, based on recordings, without active interaction with the environment, which is different from how children learn in real environments,” the authors of the research acknowledge.
Sign up for our weekly newsletter to get more English-language news coverage from EL PAÍS USA Edition
Tu suscripción se está usando en otro dispositivo
¿Quieres añadir otro usuario a tu suscripción?
Si continúas leyendo en este dispositivo, no se podrá leer en el otro.
FlechaTu suscripción se está usando en otro dispositivo y solo puedes acceder a EL PAÍS desde un dispositivo a la vez.
Si quieres compartir tu cuenta, cambia tu suscripción a la modalidad Premium, así podrás añadir otro usuario. Cada uno accederá con su propia cuenta de email, lo que os permitirá personalizar vuestra experiencia en EL PAÍS.
En el caso de no saber quién está usando tu cuenta, te recomendamos cambiar tu contraseña aquí.
Si decides continuar compartiendo tu cuenta, este mensaje se mostrará en tu dispositivo y en el de la otra persona que está usando tu cuenta de forma indefinida, afectando a tu experiencia de lectura. Puedes consultar aquí los términos y condiciones de la suscripción digital.