Can machines predict a hit song? New study finds they can and with 97% accuracy
Researchers have used AI to evaluate brain responses to music. According to their findings, this model can accurately determine which tracks trigger an emotional response and are more likely to be chart-toppers
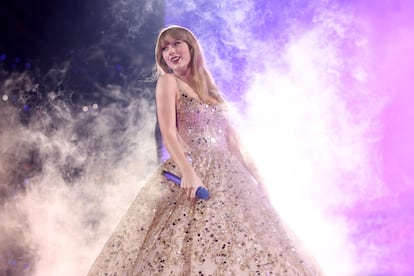
Many artists dream of creating a song that’s a hit on platforms such as Spotify or Apple Music, but less than 4% of new songs will make it onto the charts. While there’s no magic formula for making a top-charting song, a new study suggests that machine learning — an artificial intelligence technique — applied to people’s brain responses can identify songs that arouse their emotions. And these songs are usually the ones that become hits.
The study, carried out by researchers at Claremont Graduate University in the Los Angeles area, used conventional sensors — such as those found in smartwatches — to analyze human neurophysiological responses and rate a selection of songs. In the research, published in the journal Frontiers in Artificial Intelligence, 33 participants listened to 24 songs selected by staff from a streaming service. Of the selection, 13 were hits (with more than 700,000 streams) and the rest were not. The researchers measured the participants’ brain signals associated with attentional status (from dopamine release) and emotional response (linked to oxytocin). Together these neural signals were able to accurately predict behaviors after a stimulus, especially those that elicit emotional responses. In essence, it was like having a window into the mind. This window allowed researchers to study music’s effect on the brain.
Paul Zak, the lead author of the study and a professor at Claremont Graduate University, explains that people may say they like a song because of features such as rhythm or tone. But, he continues, it’s impossible to be fully aware of our intrinsic motives. “It turns out that the brain knows. Even if you cannot consciously identify it, the unconscious brain systems do know if something is good or not,” he explains.
The study showed that participants’ neurophysiological responses were able to predict which songs were the most popular, based on music market figures. A linear statistical model achieved a 69% success rate in identifying hit songs, and by applying machine learning, the researchers increased its accuracy to 97%. Even when analyzing the neural responses after just the first minute of the track, the songs were accurately classified 82% of the time.
Despite these promising results, the team acknowledges the limitations of the study, such as the relatively small number of songs used in their analysis and the lack of certain demographic groups among the participants. However, they believe that the novel methodology could be applied to other forms of entertainment, such as movies and TV shows, which could be a major game changer for the entertainment industry. For other types of content, such as audiovisuals, the data would need to be modeled differently, but the neurophysiological responses would remain the same, says Zak. “The methodology is solid, which means that it can be used over and over again, although each model will be slightly different.”
Streaming platforms often have their own models for recommending songs, but these are generally based on algorithms, expert analysis and listener behavior, such as whether a user has liked a track. Melanie Parejo, the head of music for southern and eastern Europe at Spotify, explains that the platform’s methodology employs a “wide range of learning techniques,” ranging from “collaborative filtering to reinforcement learning.”
Parejo explains that musical trends reflect Spotify’s internal factors, such as the number of streams and a song’s growth rate, as well as external factors, such as what is happening online and on TV. “There are multiple consumption signals that can contribute to the success of a song, from its growth rate to the organic consumption of the song, as well as whether users are proactively searching of if they do not skip it if it appears in a playlist. But our editorial teams also take into account the broader context, what is happening outside the platform, how it is shared on social media or if, for example, it is experiencing a resurgence thanks to a TV series,” says Parejo.
In search of a hit
If the method proposed by the U.S. researchers proves to be effective in identifying hit songs, could it perhaps help create the perfect track? Professor Zak believes it could help, but says it would be up to a musician or band to first create the song. The artist could then invite a few people to listen to the track to gauge the intensity of their emotional response. Based on this information, they could fine-tune different musical elements, be it chord changes or rhythm changes, in a bid to amplify the song’s emotional impact. “That’s the approach that some people are already starting to take today,” says Zak. However, when it comes to creating a hit song from scratch, he doesn’t believe the model is enough. “We need artists to do that initial creative work. There is no way to start at square one and artificially produce the perfect song,” he says.
Professor Sergi Jordà, who has been researching the relationship between music and technology for more than 30 years, agrees that deciphering brain signals through sensors can help optimize songs, but “is not enough to create hits.” But it could be only a matter of time before this changes. Given the rapid advancement of generative artificial intelligence and mood sensors, it is not unreasonable to think that machines could soon be creating top-charting songs.
Indeed, we are at the gates of that future. As of November 2022, Chinese streaming giant Tencent Music Entertainment has produced and released over a thousand previously unreleased songs with AI-generated voices that mimic the human voice. One song, titled Today, has been streamed 100 million times and has become the first song with AI vocals to reach this figure, according to a report by Music Business Worldwide.
Jordà points out that AI’s ability to create music from text has astounded experts. What’s more, given neural networks can create variations based on what already exists, they may become the composers of groundbreaking hits in the near future. “It is clear that, trained on big hits, they will tend to do things that resemble popular songs,” says Jordà. “This is a very dystopian future,” he continues. “It’s worrisome, and it is real.” Jordà also suggests other possibilities for AI in music, such as songs that are created in real-time and optimized according to a person’s mood.
For his part, Zak believes that the method developed by his team could benefit artists starting out in the business. “If you’re the Rolling Stones, and you’ve played at about ten thousand concerts, you already know, more or less, what’s good and what’s bad,” he says. In contrast, an amateur musician could learn from understanding that while they may like their music, their songs may not necessarily resound with others. “It’s not the only reason to create art, but if you want to create art that touches people emotionally, then it has to touch not only you, but others as well,” says Zak.
Sign up for our weekly newsletter to get more English-language news coverage from EL PAÍS USA Edition
Tu suscripción se está usando en otro dispositivo
¿Quieres añadir otro usuario a tu suscripción?
Si continúas leyendo en este dispositivo, no se podrá leer en el otro.
FlechaTu suscripción se está usando en otro dispositivo y solo puedes acceder a EL PAÍS desde un dispositivo a la vez.
Si quieres compartir tu cuenta, cambia tu suscripción a la modalidad Premium, así podrás añadir otro usuario. Cada uno accederá con su propia cuenta de email, lo que os permitirá personalizar vuestra experiencia en EL PAÍS.
En el caso de no saber quién está usando tu cuenta, te recomendamos cambiar tu contraseña aquí.
Si decides continuar compartiendo tu cuenta, este mensaje se mostrará en tu dispositivo y en el de la otra persona que está usando tu cuenta de forma indefinida, afectando a tu experiencia de lectura. Puedes consultar aquí los términos y condiciones de la suscripción digital.