The dangers of letting algorithms make decisions for you
Experts warn that AI systems used by recruiters, banks and even judges learn from data that can contain an undesired bias
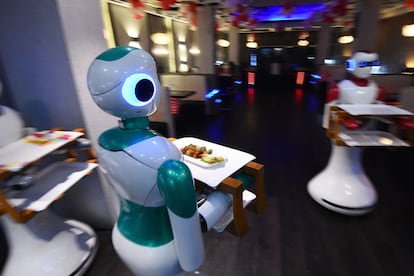
In 2014, Amazon developed an artificial intelligence (AI) recruitment tool that began to discriminate against female job applicants. A year later, a user of Google Photos discovered that the program was labeling his black friends as gorillas. And in 2018 it emerged that an algorithm that analyzed the risk of recidivism by a million US defendants made as many mistakes as any human being with no training in criminal justice.
Decisions that were once made by human beings are now being made by AI systems. Some programs deal with hiring, others with loan approvals, medical diagnoses and even court rulings. But there is a risk involved, because the data used to “train” the algorithms is itself conditioned by our own knowledge and prejudices.
The data is a reflection of reality. If reality is prejudiced, so is the data
Richard Benjamins, Telefónica
“The data is a reflection of reality. If reality is prejudiced, so is the data,” explains Richard Benjamins, the Big Data and AI ambassador at Telefónica, a global telco and tech company, in a telephone conversation with EL PAÍS. To prevent an algorithm from discriminating against certain groups, he says, it is necessary to check that the training data does not contain a bias, and to analyze the false positive and negative ratios. “It is much more serious to have an algorithm that discriminates in an undesired way in the fields of law, loans or school admissions than in the fields of movie recommendations or advertising.”
Isabel Fernández, a managing director of applied intelligence at consulting firm Accenture, brings up the example of automatic loan grants. “Imagine that in the past, most of the applicants were men, and the few women who were approved for a loan had to overcome such stringent criteria that they all repaid their debt. If we used this data and nothing else, the system would conclude that women are better at paying back than men, which is merely a reflection of a past prejudice.”
Yet on many occasions it is women who are harmed by this kind of bias. “Algorithms are generally developed because a group of mostly white men between the ages of 25 and 50 have decided so at a meeting. With this kind of starting point, it is difficult to include the opinion or perception of minority groups or of the other 50% of the population, which is made up of women,” says Nerea Luis Mingueza, a robotics and AI researcher at Carlos III University who holds that underrepresented groups will always be affected to a greater degree by technological products. “For instance, the voices of women and children fail more often in voice recognition systems.”
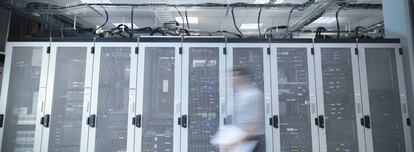
Minorities are more likely to feel the effects of this bias for statistical reasons, says José María Lucia, who heads the AI and data analysis center at EY Wavespace: “The number of available cases for training is going to be lower,” he notes. “And any groups who have suffered discrimination of any type in the past are open to this, because by using historical data we could be unwittingly including this bias in the training.”
This is the case with the African-American population in the United States, according to Juan Alonso, a senior manager at Accenture. “It’s been proven that if caught over the same kind of misdemeanor, such as smoking a joint in public or possessing small amounts of marijuana, a white person will not get arrested but a black person will.” This means that there is a higher percentage of black people on the database, and an algorithm that is trained with this information will incorporate a racist bias.
Sources at Google explain that it is essential to “be very careful” about giving decision-making power to a machine-learning system. “Artificial intelligence produces answers based on existing data, and so humans must recognize that they won’t necessarily provide impeccable results.”
Algorithms are generally developed because a group of mostly white men between the ages of 25 and 50 have so decided at a meeting
Nerea Luis Mingueza, Carlos III University
Machines often end up being a black box filled with secrets that are undecipherable even to their own developers, who are unable to figure out what path the model followed to reach a certain conclusion. Alonso holds that “normally, when you are tried, you get an explanation for the decision in a ruling. But the problem is that this type of algorithm is opaque. It’s like being in the presence of an oracle who is going to hand down a verdict.”
“Imagine you are going to an open-air festival and when you reach the front row, the security team kicks you out without any kind of explanation. You would be incensed. But if you are told that the first row is reserved for people in wheelchairs, you would not be angry about moving back. It’s the same with these algorithms: if we don’t know what’s going on, it can generate a feeling of dissatisfaction,” explains Alonso.
To end this dilemma, researchers working on machine learning advocate greater transparency and providing explanations for training models. Major tech companies like Microsoft defend a set of principles for a responsible use of AI, and they are sponsoring initiatives to try to crack open the black box of algorithms and explain why decisions get made the way they are.
Telefonica is organizing a Challenge for a Responsible Use of AI at its Big Data business unit, LUCA, with the aim of creating new tools to detect undesired bias. Accenture has developed AI Fairness and IBM has also come up with its own bias-detection tool. For Francesca Rossi, director of ethics and AI at IBM, the key lies in making AI systems transparent and trustworthy. “People have the right to ask how an intelligent system suggests certain decisions over others, and companies have a duty to help people understand the decision-making process.”
English version by Susana Urra.