Ethical machines? How to teach morals to computers by translating deontology into numbers
In the midst of the debate about the risks of artificial intelligence, formulas are being sought to make these systems prioritize some decisions over others, utilizing techniques such as reinforcement learning
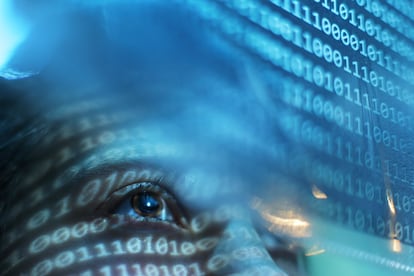
There are three basic elements in the mathematical formula for teaching a code of ethics to machines. This does not differ much from the ethical cocktail that people live by: action, value and rules make up the trinity with which researchers play to establish limitations that control the behavior of artificial intelligences.
For people, value is equivalent to a sort of commonly accepted social norm: we know that lying is a morally reprehensible action. And rules help formalize the idea of value in a legal code. “Rules prohibit, just as smoking is prohibited in closed spaces, but value also helps you promote good actions, such as making a donation or being kind,” explains Maite López-Sánchez, an artificial intelligence (AI) researcher and professor at the University of Barcelona (Spain) who is working on systems to introduce ethical principles in AI systems.
People learn this structure, which serves to guide our behavior during the socialization process, but in machines everything must be translated into numbers and mathematical functions. The final goal is to provide a framework for the actions: “Machines are very integrated into society and end up making decisions that affect us. It would be desirable for these decisions to be aligned with what we understand to be correct, that they integrate well, socially,” says the researcher.
López-Sánchez resorts to the most basic terms to explain the need to have ethical machines: “I can have a self-driving car and, if I tell it to take me to work, it would take the route that was most efficient or fastest. We are very clear that I want to get to work, but I also don’t want to run over anyone. It wouldn’t be morally right.” However, casuistry goes far beyond the extremes. “There are many aspects to take into account to drive correctly. It’s not just about not breaking the rules; it’s about doing things well, like yielding to a pedestrian, maintaining a safe distance or not being aggressive with the horn,” adds the researcher.
Ethics in artificial intelligence also serves to promote equal treatment. “If it is a decision-making system for granting health insurance, what we want is an algorithm that has no bias, that treats all the people it assesses equally,” says López-Sánchez.
In recent years, all kinds of algorithmic biases have come to light. A system developed by Amazon to select candidates for a job favored men’s résumés over women’s; it did that because it was trained on a majority of male résumés, and there was no way to correct this deviation. Another algorithm, this one used by the U.S. health care system, assigned white people a greater level of risk than that of Black people, thus giving them priority in medical care.
In addition, autonomous systems deal with issues related to intellectual property or the use of private data. One formula to avoid these shortcomings is to set self-limitations in the design of the algorithm. Ana Cuevas, teacher in the area of logic and philosophy of science at the University of Salamanca (Spain), advocates for this proactive approach: “We don’t have to wait for things to happen to analyze the possible risks; we have to start from the assumption that before we create an artificial intelligence system we have to think about what type of system we want to create to avoid certain undesirable results.”
Ethics in machine language
The introduction of an ethical framework into machines is a relatively new endeavor. The scientific community has primarily approached it from a theoretical standpoint, but it is not as common to shift into action to specify values in figures and moral teachings in engineering. In the research group led by Sánchez-López, WAI, at the University of Barcelona, they explore this field experimentally.
These researchers link the concepts of value and action in system design. “We have mathematical functions that tell us that for a certain value, a certain action of the machine is considered positive or negative,” says López-Sánchez. Thus, in the example of a self-driving car, smooth driving on a winding road will be considered positive in terms of the value of safety. However, if viewed from the perspective of the value of kindness to other drivers, the vehicle might decide to increase its speed if it notices that it is slowing other cars down.
In this specific case, there would be a conflict between values, which would be resolved through deliberation. Preferences are established beforehand, indicating which values to prioritize. The entire set comprises interconnected formulas, which must also take into account the variable of the rule. “There is another function that stipulates that a rule promotes a value,” notes the researcher. “And we also have functions that observe how a rule assesses the action, and also how the value assesses that action.” It is a complex system in which feedback is key.
When López-Sánchez talks about assessing, she is referring directly to machine learning. One of the ways machines learn is through reinforcement, like humans, who do the right thing because we are rewarded, and avoid wrongdoing because we are punished. This mechanism also applies to artificial intelligence.
“Rewards are numbers. We reward them with positive numbers and punish them with negative numbers,” explains the WAI researcher. “Machines try to score as many points as possible. So, the machine will try to behave well if I give it positive numbers when it does things right. And if I punish it and deduct points when it behaves badly, it will try not to do so.” Just like children in school, scoring serves educational purposes.
However, many issues still need to be sorted out, starting with something as simple as deciding which values to introduce into machines. “Ethics develops in very different ways. In some cases, we may need to make utilitarian calculations, minimizing risks or damages,” says Cuevas. “Other times we may need to use stronger deontological codes: for example, establishing that a system cannot lie. Each system needs to incorporate certain values, and for this, there must be community and social agreement.”
In López-Sánchez’s laboratory, they scrutinize sociological studies to find common values among people and across different cultures. They also use international references like the U.N. Universal Declaration of Human Rights. However, at a global level, some aspects will be more challenging to agree on. “The limitations of machines will have their boundaries. The European Union, for instance, has a way of doing things, and the United States has another,” emphasizes Cuevas.
Sign up for our weekly newsletter to get more English-language news coverage from EL PAÍS USA Edition
Tu suscripción se está usando en otro dispositivo
¿Quieres añadir otro usuario a tu suscripción?
Si continúas leyendo en este dispositivo, no se podrá leer en el otro.
FlechaTu suscripción se está usando en otro dispositivo y solo puedes acceder a EL PAÍS desde un dispositivo a la vez.
Si quieres compartir tu cuenta, cambia tu suscripción a la modalidad Premium, así podrás añadir otro usuario. Cada uno accederá con su propia cuenta de email, lo que os permitirá personalizar vuestra experiencia en EL PAÍS.
En el caso de no saber quién está usando tu cuenta, te recomendamos cambiar tu contraseña aquí.
Si decides continuar compartiendo tu cuenta, este mensaje se mostrará en tu dispositivo y en el de la otra persona que está usando tu cuenta de forma indefinida, afectando a tu experiencia de lectura. Puedes consultar aquí los términos y condiciones de la suscripción digital.